CAVE LAB
Complex data can be difficult to visualize and analyze. Working in cross-functional teams also adds a layer of complexity to making decisions from big data analysis. The CAVE Lab uses interactive visualization to improve data visibility, data analysis, and decision making for supply chain and logistics challenges.
We aim to harness the power of AI and machine learning in combination with state-of-the-art optimization methods to tackle the most significant real-world challenges facing the logistics industry today.
Visualize Complex data with the MIT CAVE Lab
Complex data can be difficult to visualize and analyze. Working in cross-functional teams also adds a layer of complexity to making decisions from big data analysis. The CAVE Lab uses interactive visualization to improve data visibility, data analysis, and decision-making for supply chain and logistics challenges.
Our Expertise & Research
The CAVE lab provides students, researchers, and decision-makers with a more intuitive understanding of and access to quantitative methods to support strategic design, tactical planning, and operational decision problems in the supply chain and logistics domain and related fields.
Based on a newly created physical lab space at MIT CTL equipped with state-of-the-art visualization technology, the lab is developing interactive visual interfaces to data and analytical tools, addressing complex supply chain and logistics problems.
Development, improvement, and application of traditional quantitative methods in the supply chain, logistics, and transportation decision making (network design, distribution systems, inventory management, risk management, etc.)
Adaptation and application of advanced data science methods (machine learning, network science, etc.) to large and diverse datasets to characterize, understand, predict, and improve the performance of complex supply networks, transportation and logistics systems
Behavioral analysis of human decision making in supply chain management, transportation and logistics in light of interactive visualization being used as a tool to communicate, analyze, and manipulate context- and problem-related information
Innovative Education
The lab provides a unique environment for students from all programs of MIT at all levels of seniority to understand and experiment with quantitative methods of supply chain, transportation, and logistics decision methods. From studying the principles of data science, optimization or simulation through interactive demo applications, to developing course projects or thesis projects that combine our domain with interactive visual interfaces, it provides students an intuitive, open source environment to understand the theory, apply it to real data, and implement usable demos and applications.
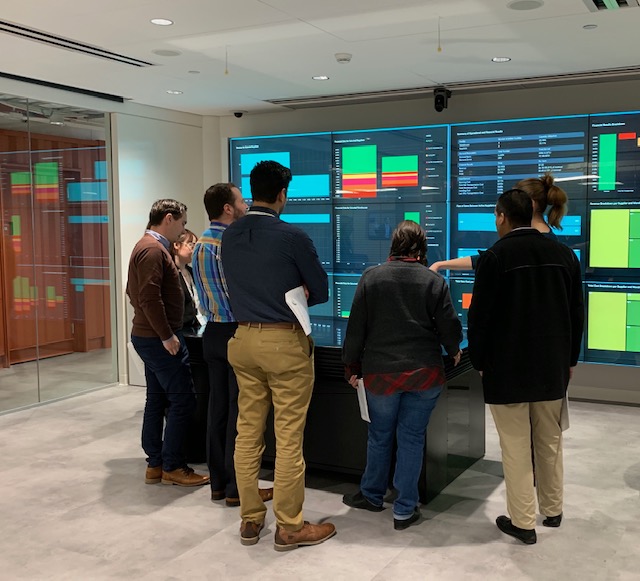
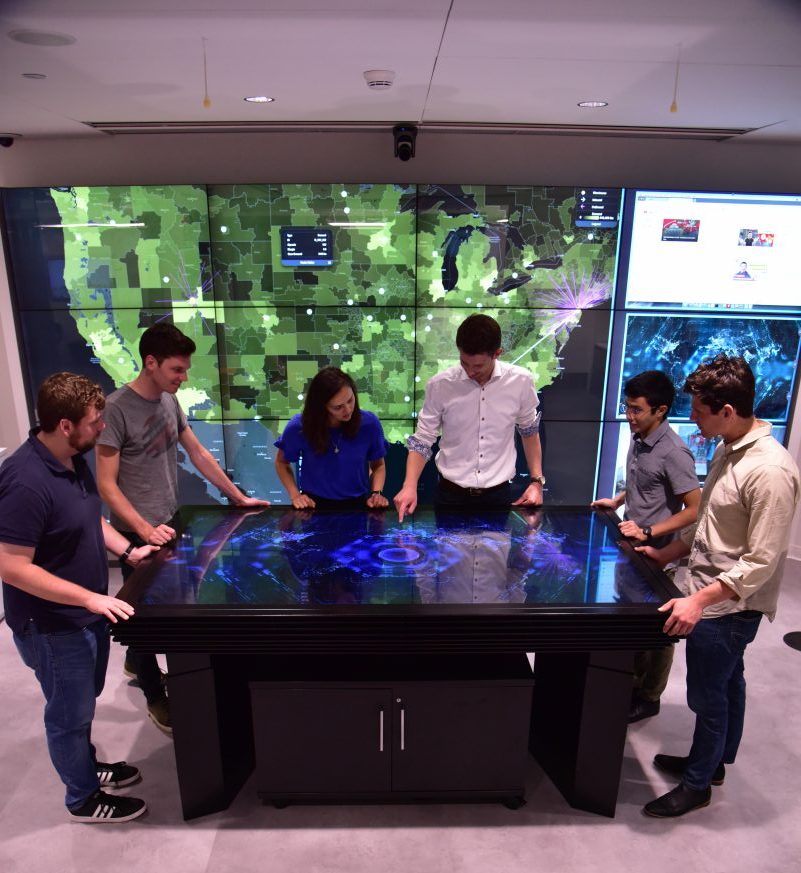
Smarter Decision Making
Together with MIT CTL’s corporate partners, the lab can be leveraged as an interactive decision-making space. By co-developing interactive visual analytics applications addressing specific, data-driven decision problems of our partners and presenting them in a way that optimally leverages human perception and cognition, we aim to promote the effective future use of data and analytics by corporate decision-makers at all levels.
Corporate engagement with the lab can be based on dedicated research and development projects and may entail interactive decision making and data analytics workshops with employees, customers, suppliers, or peers of the sponsoring partner at the lab.